Digital Decider: The Power (Biases?) of AI in Job Screening
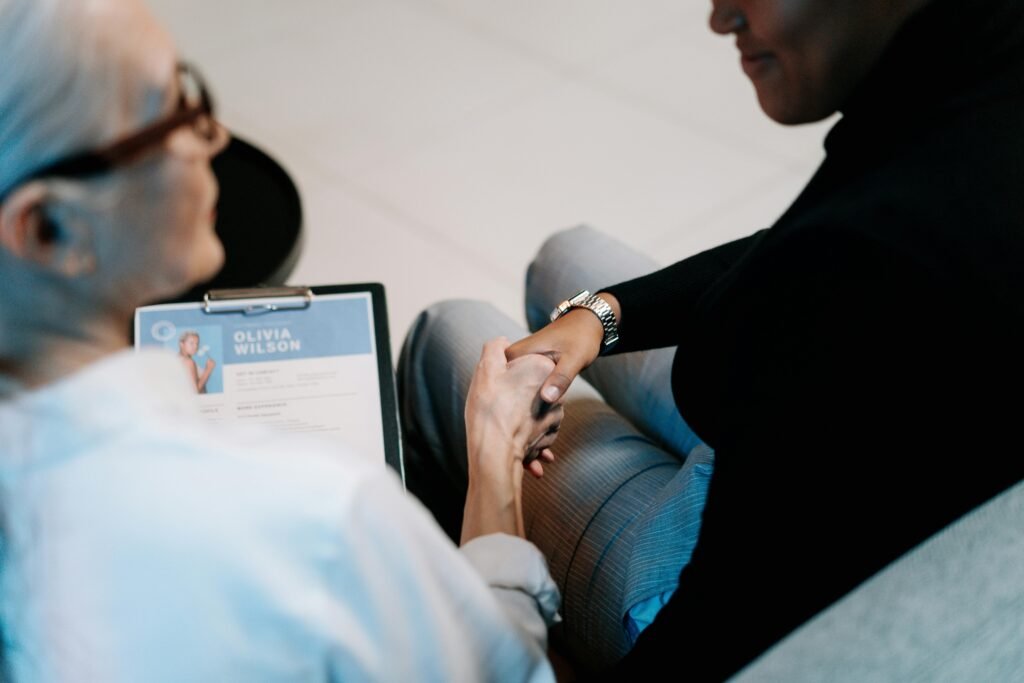
In an era where a staggering 70% of resumes are sifted by AI before reaching human hands, the question of bias in these digital gatekeepers looms large. Artificial Intelligence (AI) in job screening is transforming hiring practices, but this revolution brings with it a host of potential biases that could shape the future workforce.
AI Screening: A Double-Edged Sword
While AI applications like Applicant Tracking Systems (ATS), used by LinkedIn and Indeed, efficiently process vast volumes of resumes, they’re not free from prejudice. These systems, programmed with certain keywords and criteria, can inadvertently favor candidates based on their similarity to existing employees. This practice, known as “mirroring,” risks perpetuating a lack of diversity in workplaces.
Predictive Analytics: Reflecting Historical Biases
Predictive analytics tools, such as those employed by IBM’s Watson, assess candidates’ future performance based on historical data. However, this data can reflect historical biases in hiring, potentially disadvantaging certain groups. For instance, if historical hiring trends show a preference for candidates from specific universities, the AI might unjustly favor applicants from those institutions.
Customized AI Algorithms and Industry-Specific Risks
In tech sectors, where AI focuses on technical skills and coding proficiency, there’s a risk of gender bias, given the historically male-dominated nature of the field. Similarly, in customer service, AI assessments of communication skills might favor certain linguistic styles, potentially discriminating against non-native speakers or those with different cultural communication norms.
Natural Language Processing: Subtleties of Bias
Natural Language Processing (NLP) applications like XOR and Mya, which analyze responses for linguistic nuances, can also inadvertently propagate biases. These systems may favor certain speech patterns or idiomatic expressions, disadvantaging candidates from diverse linguistic backgrounds.
AI and Diversity: A Work in Progress
Efforts like LinkedIn’s AI fairness toolkit aim to combat bias by adjusting algorithms to be more inclusive. However, the effectiveness of these tools is contingent on continuous monitoring and updating to ensure they adapt to evolving understandings of fairness and diversity.
The integration of AI in job screening, while efficient, demands a vigilant approach to prevent the perpetuation of biases. As AI technologies become more sophisticated, the need for ethical programming and diverse data sets becomes crucial. The future of equitable hiring depends on our ability to harness AI not just as a tool for efficiency but as an instrument for inclusive progress.